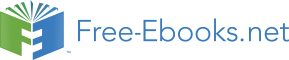

1. THE DISCRETE NOISELESS CHANNEL
Teletype and telegraphy are two simple examples of a discrete channel for transmitting information. Gen-
erally, a discrete channel will mean a system whereby a sequence of choices from a finite set of elementary
symbols S 1
Sn can be transmitted from one point to another. Each of the symbols Si is assumed to have
;
:
:
:
;
a certain duration in time ti seconds (not necessarily the same for different Si, for example the dots and dashes in telegraphy). It is not required that all possible sequences of the Si be capable of transmission on the system; certain sequences only may be allowed. These will be possible signals for the channel. Thus
in telegraphy suppose the symbols are: (1) A dot, consisting of line closure for a unit of time and then line
open for a unit of time; (2) A dash, consisting of three time units of closure and one unit open; (3) A letter space consisting of, say, three units of line open; (4) A word space of six units of line open. We might place the restriction on allowable sequences that no spaces follow each other (for if two letter spaces are adjacent, it is identical with a word space). The question we now consider is how one can measure the capacity of
such a channel to transmit information.
In the teletype case where all symbols are of the same duration, and any sequence of the 32 symbols
is allowed the answer is easy. Each symbol represents five bits of information. If the system transmits n
symbols per second it is natural to say that the channel has a capacity of 5 n bits per second. This does not mean that the teletype channel will always be transmitting information at this rate — this is the maximum
possible rate and whether or not the actual rate reaches this maximum depends on the source of information
which feeds the channel, as will appear later.
In the more general case with different lengths of symbols and constraints on the allowed sequences, we
make the following definition:
Definition: The capacity C of a discrete channel is given by
log N T
C
Lim
=
T
∞
T
!
where N T is the number of allowed signals of duration T .
It is easily seen that in the teletype case this reduces to the previous result. It can be shown that the limit in question will exist as a finite number in most cases of interest. Suppose all sequences of the symbols
S 1
Sn are allowed and these symbols have durations t 1
tn. What is the channel capacity? If N t
;
:
:
:
;
;
:
:
:
;
represents the number of sequences of duration t we have
N t
N t
t 1
N t
t 2
N t
tn
=
,
+
,
+
+
,
:
The total number is equal to the sum of the numbers of sequences ending in S 1 S 2
Sn and these are
;
;
:
:
:
;
N t
t 1 N t
t 2
N t
tn , respectively. According to a well-known result in finite differences, N t
,
;
,
;
:
:
:
;
,
is then asymptotic for large t to Xt where X
0
0 is the largest real solution of the characteristic equation:
X t
t
tn
,
1
X , 2
X ,
1
+
+
+
=
3
and therefore
C
log X 0
=
:
In case there are restrictions on allowed sequences we may still often obtain a difference equation of this
type and find C from the characteristic equation. In the telegraphy case mentioned above
N t
N t
2
N t
4
N t
5
N t
7
N t
8
N t
10
=
,
+
,
+
,
+
,
+
,
+
,
as we see by counting sequences of symbols according to the last or next to the last symbol occurring.
Hence C is
log
2
4
5
7
8
10
0 where
0 is the positive root of 1
. Solving this we find
,
=
+
+
+
+
+
C
0 539.
=
:
A very general type of restriction which may be placed on allowed sequences is the following: We
imagine a number of possible states a 1 a 2
am. For each state only certain symbols from the set S 1
Sn
;
;
:
:
:
;
;
:
:
:
;
can be transmitted (different subsets for the different states). When one of these has been transmitted the
state changes to a new state depending both on the old state and the particular symbol transmitted. The
telegraph case is a simple example of this. There are two states depending on whether or not a space was
the last symbol transmitted. If so, then only a dot or a dash can be sent next and the state always changes.
If not, any symbol can be transmitted and the state changes if a space is sent, otherwise it remains the same.
The conditions can be indicated in a linear graph as shown in Fig. 2. The junction points correspond to the
DASH
DOT
DOT
LETTER SPACE
DASH
WORD SPACE
Fig. 2 — Graphical representation of the constraints on telegraph symbols.
states and the lines indicate the symbols possible in a state and the resulting state. In Appendix 1 it is shown that if the conditions on allowed sequences can be described in this form C will exist and can be calculated in accordance with the following result:
s
Theorem 1: Let b be the duration of the s th symbol which is allowable in state i and leads to state j.
i j
Then the channel capacity C is equal to log W where W is the largest real root of the determinant equation:
∑
s
W b
,
i j
i j
0
,
=
s
where i j
1 if i
j and is zero otherwise.
=
=
For example, in the telegraph case (Fig. 2) the determinant is:
1
W 2
4
,
W ,
,
+
0
W 3
6
2
4
=
:
,
W ,
W ,
W ,
1
+
+
,
On expansion this leads to the equation given above for this case.
2. THE DISCRETE SOURCE OF INFORMATION
We have seen that under very general conditions the logarithm of the number of possible signals in a discrete
channel increases linearly with time. The capacity to transmit information can be specified by giving this
rate of increase, the number of bits per second required to specify the particular signal used.
We now consider the information source. How is an information source to be described mathematically,
and how much information in bits per second is produced in a given source? The main point at issue is the
effect of statistical knowledge about the source in reducing the required capacity of the channel, by the use
4
of proper encoding of the information. In telegraphy, for example, the messages to be transmitted consist of
sequences of letters. These sequences, however, are not completely random. In general, they form sentences
and have the statistical structure of, say, English. The letter E occurs more frequently than Q, the sequence
TH more frequently than XP, etc. The existence of this structure allows one to make a saving in time (or
channel capacity) by properly encoding the message sequences into signal sequences. This is already done
to a limited extent in telegraphy by using the shortest channel symbol, a dot, for the most common English
letter E; while the infrequent letters, Q, X, Z are represented by longer sequences of dots and dashes. This
idea is carried still further in certain commercial codes where common words and phrases are represented
by four- or five-letter code groups with a considerable saving in average time. The standardized greeting
and anniversary telegrams now in use extend this to the point of encoding a sentence or two into a relatively
short sequence of numbers.
We can think of a discrete source as generating the message, symbol by symbol. It will choose succes-
sive symbols according to certain probabilities depending, in general, on preceding choices as well as the
particular symbols in question. A physical system, or a mathematical model of a system which produces
such a sequence of symbols governed by a set of probabilities, is known as a stochastic process.3 We may
consider a discrete source, therefore, to be represented by a stochastic process. Conversely, any stochastic
process which produces a discrete sequence of symbols chosen from a finite set may be considered a discrete
source. This will include such cases as:
1. Natural written languages such as English, German, Chinese.
2. Continuous information sources that have been rendered discrete by some quantizing process. For
example, the quantized speech from a PCM transmitter, or a quantized television signal.
3. Mathematical cases where we merely define abstractly a stochastic process which generates a se-
quence of symbols. The following are examples of this last type of source.
(A) Suppose we have five letters A, B, C, D, E which are chosen each with probability .2, successive
choices being independent. This would lead to a sequence of which the following is a typical
example.
B D C B C E C C C A D C B D D A A E C E E A
A B B D A E E C A C E E B A E E C B C E A D.
This was constructed with the use of a table of random numbers.4
(B) Using the same five letters let the probabilities be .4, .1, .2, .2, .1, respectively, with successive
choices independent. A typical message from this source is then:
A A A C D C B D C E A A D A D A C E D A
E A D C A B E D A D D C E C A A A A A D.
(C) A more complicated structure is obtained if successive symbols are not chosen independently
but their probabilities depend on preceding letters. In the simplest case of this type a choice
depends only on the preceding letter and not on ones before that. The statistical structure can
then be described by a set of transition probabilities pi j , the probability that letter i is followed by letter j. The indices i and j range over all the possible symbols. A second equivalent way of specifying the structure is to give the “digram” probabilities p i j , i.e., the relative frequency of
;
the digram i j. The letter frequencies p i , (the probability of letter i), the transition probabilities 3See, for example, S. Chandrasekhar, “Stochastic Problems in Physics and Astronomy,” Reviews of Modern Physics, v. 15, No. 1, January 1943, p. 1.
4Kendall and Smith, Tables of Random Sampling Numbers, Cambridge, 1939.
5
pi j and the digram probabilities p i j are related by the following formulas:
;
p i
∑ p i j ∑ p j i ∑ p j pj i
=
;
=
;
=
j
j
j
p i j
p i pi j
;
=
∑ pi j ∑ p i ∑ p i j 1
=
=
;
=
:
j
i
i j
;
As a specific example suppose there are three letters A, B, C with the probability tables:
pi j
j
i
p i
p i j
j
;
A
B
C
A
B
C
A
0
4
1
A
9
A
0
4
1
5
5
27
15
15
i
B
1
1
0
B
16
i
B
8
8
0
2
2
27
27
27
C
1
2
1
C
2
C
1
4
1
2
5
10
27
27
135
135
A typical message from this source is the following:
A B B A B A B A B A B A B A B B B A B B B B B A B A B A B A B A B B B A C A C A B
B A B B B B A B B A B A C B B B A B A.
The next increase in complexity would involve trigram frequencies but no more. The choice of
a letter would depend on the preceding two letters but not on the message before that point. A
set of trigram frequencies p i j k or equivalently a set of transition probabilities pi j k would
;
;
be required. Continuing in this way one obtains successively more complicated stochastic pro-
cesses. In the general n-gram case a set of n-gram probabilities p i 1 i 2
in or of transition
;
;
:
:
:
;
probabilities pi
i
is required to specify the statistical structure.
1 i
i
n
;
2;:::; n 1
,
(D) Stochastic processes can also be defined which produce a text consisting of a sequence of
“words.” Suppose there are five letters A, B, C, D, E and 16 “words” in the language with
associated probabilities:
.10 A
.16 BEBE
.11 CABED
.04 DEB
.04 ADEB
.04 BED
.05 CEED
.15 DEED
.05 ADEE
.02 BEED
.08 DAB
.01 EAB
.01 BADD
.05 CA
.04 DAD
.05 EE
Suppose successive “words” are chosen independently and are separated by a space. A typical
message might be:
DAB EE A BEBE DEED DEB ADEE ADEE EE DEB BEBE BEBE BEBE ADEE BED DEED
DEED CEED ADEE A DEED DEED BEBE CABED BEBE BED DAB DEED ADEB.
If all the words are of finite length this process is equivalent to one of the preceding type, but
the description may be simpler in terms of the word structure and probabilities. We may also
generalize here and introduce transition probabilities between words, etc.
These artificial languages are useful in constructing simple problems and examples to illustrate vari-
ous possibilities. We can also approximate to a natural language by means of a series of simple artificial
languages. The zero-order approximation is obtained by choosing all letters with the same probability and
independently. The first-order approximation is obtained by choosing successive letters independently but
each letter having the same probability that it has in the natural language.5 Thus, in the first-order ap-
proximation to English, E is chosen with probability .12 (its frequency in normal English) and W with
probability .02, but there is no influence between adjacent letters and no tendency to form the preferred
5Letter, digram and trigram frequencies are given in Secret and Urgent by Fletcher Pratt, Blue Ribbon Books, 1939. Word frequencies are tabulated in Relative Frequency of English Speech Sounds, G. Dewey, Harvard University Press, 1923.
6
digrams such as TH, ED, etc. In the second-order approximation, digram structure is introduced. After a
letter is chosen, the next one is chosen